Calgary ML Lab
The Calgary Machine Learning Lab is a research group led by Yani Ioannou within the Schulich School of Engineering at the University of Calgary. The lab has a research focus on improving Deep Neural Network (DNN) training and models. Topics of research include: Sparse Neural Network Training, Bias and Robustness of Efficient Deep Learning methods and Efficient Inference with Large Language Models.
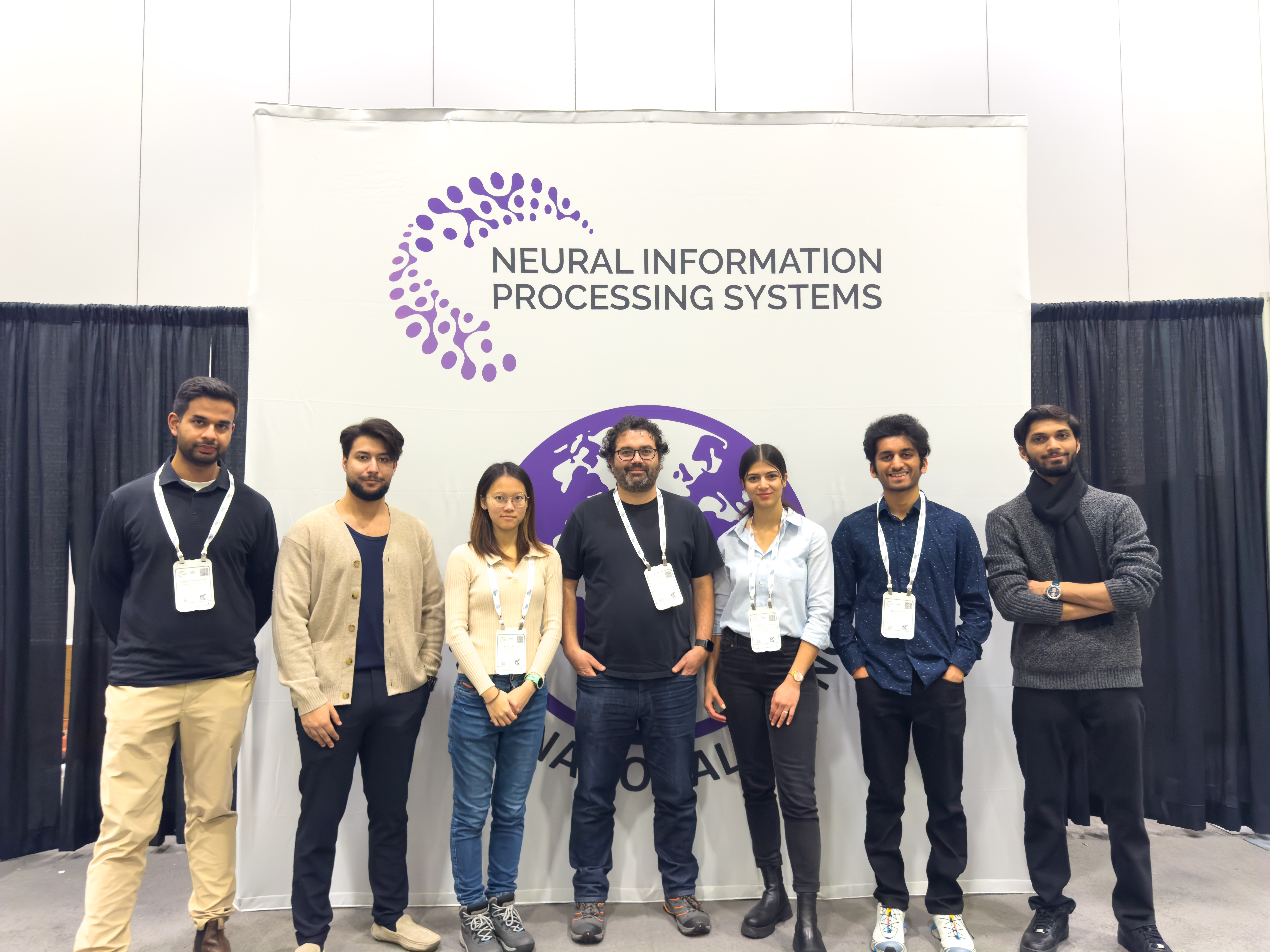
NeurIPS 2024, Vancouver, BC, Canada (December 2024)
news
Dec 5, 2024 | The Calgary Machine Learning Lab, will have 5 different works being presented by 6 students across the Neural Information Processing Systems (NeurIPS) 2024 workshops (UniReps, WiML, MusiML) and main conference! Please see our full conference schedule here for details. |
---|---|
Jul 24, 2024 | Adnan was recently awarded the NSERC Doctoral Fellowship (120,000 CAD for the next three years). The program provides financial support to high-calibre PhD students across Canada. |
Jul 2, 2024 | Mike Lasby was recently awarded the Faculty of Graduate Studies Doctoral Scholarship which recognizes his research contributions and academic achievements to date. Mike also recently passed his PhD Candidacy examination which puts him one step closer towards graduation! |
Apr 1, 2024 | CML was awarded 24 RGU (approx. 6 A100-years) of GPU compute in the Digital Research Alliance of Canada Resources for Research Groups (RRG) 2024 competition, to support our research. The RRG program provides access to a large-scale GPU clusters for academic research groups in Canada. |
Mar 1, 2024 | Muhammad Athar Ganaie was awarded a Mitacs Globalink Graduate Fellowship, to support his MSc research at the University of Calgary. The fellowship will support his research on the development of sparse neural networks for deep reinforcment learning. |
Jan 16, 2024 | Mike Lasby’s collaborative work with researchers at Google, MIT and the Vector Institute, “Dynamic Sparse Training with Structured Sparsity” (Lasby et al., 2024), was accepted at ICLR 2024! DST methods learn state-of-the-art sparse masks, but accelerating DNNs with unstructured masks is difficult. SRigL learns structured masks, improving real-world CPU/GPU timings! |
selected publications and pre-prints
- Learning Parameter Sharing with Tensor Decompositions and SparsityarXiv preprint arXiv:2411.09816 2024arXiv preprint
- What is Left After Distillation? How Knowledge Transfer Impacts Fairness and BiasarXiv preprint arXiv:2410.08407 2024arXiv preprint
-
- Winning Tickets from Random Initialization: Aligning Masks for Sparse TrainingIn 2nd Workshop on Unifying Representations in Neural Models (UniReps), NeurIPS 2024 Workshops, Vancouver, BC, Canada 2024
- Dynamic Sparse Training with Structured SparsityIn International Conference on Learning Representations (ICLR), Vienna, Austria 2024
- Gradient Flow in Sparse Neural Networks and How Lottery Tickets WinIn Proceedings of the 36th AAAI Conference on Artificial Intelligence, Vancouver, BC, Canada Feb 2022